Natural language processing (NLP), a branch of artificial intelligence, has experienced remarkable advancements in recent years, revolutionizing various industries and propelling technological development. NLP empowers computers to understand and interpret written and spoken language, mirroring human comprehension of linguistic meaning.
In the realm of AI, data annotation serves as the fundamental building block, providing labeled datasets that facilitate the training and fine-tuning of machine learning models. While text annotation has long been a crucial component of NLP, audio annotation has emerged as an indispensable process in the development and refinement of natural language understanding.
This article aims to equip you with comprehensive knowledge of audio annotation. By delving into the practical aspects of audio annotation, we will empower you to grasp the intricacies involved in this crucial step of NLP development.
What is audio annotation?
Audio annotation is the process of adding labels or annotations to audio data in order to provide context, structure, and meaning to the information contained within the audio. It involves identifying and marking specific elements or segments within the audio, such as speech, language, emotions, speaker identities, background sounds, and other relevant attributes.
Audio annotation is crucial in various applications, particularly in the field of natural language processing (NLP). By annotating audio data, researchers, data scientists, or annotators can create labeled datasets that serve as training material for machine learning models. These annotations help the models understand and interpret spoken language, enabling them to perform tasks like speech recognition, sentiment analysis, speaker identification, and more.
The process of audio annotation typically involves skilled human annotators who listen to the audio recordings and perform the annotation task based on predetermined guidelines or criteria. They may transcribe spoken words into written text, label emotions or sentiment expressed in the audio, identify different speakers, mark specific events or actions, or apply other relevant tags to capture the desired information.
What are the types of audio annotation?
There are several types of audio annotation techniques that serve different purposes in analyzing and understanding audio data. The main techniques of audio annotation include:
- Speaker Diarization:
Speaker diarization involves analyzing the audio stream to identify and classify individual speakers’ voices in a recording. This technique helps separate and distinguish different speakers, enabling tasks such as speaker identification, tracking changes in speakers, or extracting individual speaker-specific information.
- Audio Transcription:
Audio transcription is the process of converting spoken language from audio format into written text. It involves listening to the audio and transcribing the speech accurately. Audio transcription enhances the accessibility and searchability of audio content, making it easier to analyze and understand the spoken words within the audio.
- Emotion Recognition:
Emotion recognition focuses on capturing and identifying the emotions expressed by speakers in the recorded audio. By analyzing various acoustic features such as tone, pitch, rhythm, and overall voice characteristics, emotion recognition techniques can detect and classify emotions like happiness, sadness, anger, or neutral states. This information can be valuable in applications like sentiment analysis, customer feedback analysis, or emotion-aware systems.
- Audio Classification:
Audio classification involves categorizing audio data into different predefined categories based on its content. There are several subcategories of audio classification:
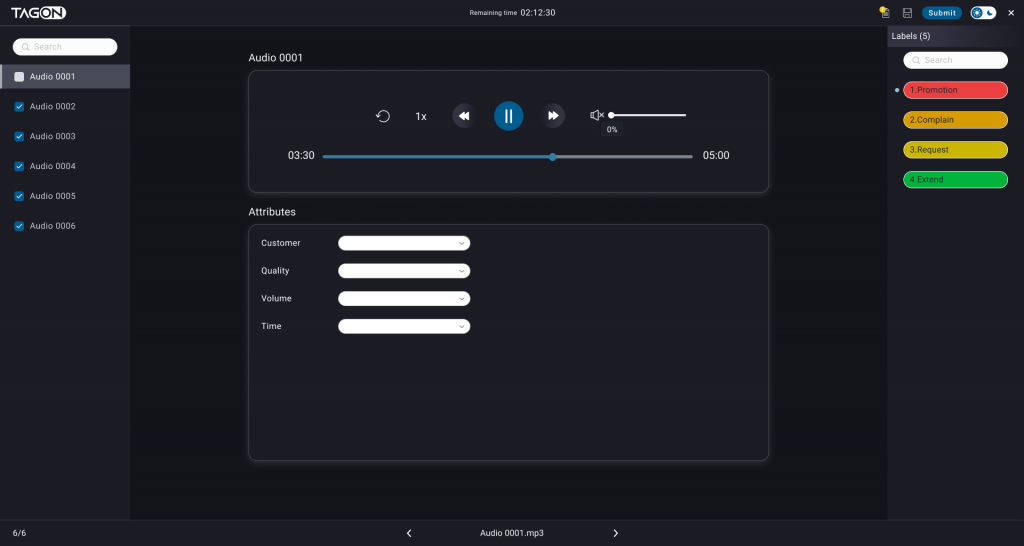
Audio Classification tool on TagOn
Acoustic Data Classification:
Acoustic data classification focuses on categorizing audio based on the acoustic characteristics of the recording environment. This can include distinguishing between different environments like schools, hospitals, streets, or indoor versus outdoor settings.
Environmental Sound Classification:
Environmental sound classification involves identifying and classifying specific sounds within the audio that are associated with the environment. This can include differentiating human voices, car horns, animal sounds, or other environmental noises.
Music Classification:
Music classification aims to categorize audio recordings based on musical content. This can involve identifying different musical instruments, genres, styles, or even recognizing specific artists or singers.
Natural Language Utterance Classification:
Natural language utterance classification focuses on classifying spoken language based on dialects, pronunciations, tones, or other linguistic features. This type of classification is often employed in applications like chatbots or virtual assistants to improve natural language understanding and response generation.
These various types of audio annotation techniques provide valuable insights into the content, structure, and context of audio data, enabling more accurate analysis, interpretation, and utilization of the recorded audio in a wide range of applications.
Why is audio annotation important ?
Audio annotation plays a crucial role in various domains and applications due to its importance and the value it brings to the analysis and understanding of audio data. Here are some key reasons why audio annotation is important:
- Enhancing Natural Language Processing (NLP):
Audio annotation contributes to the development of NLP systems by providing labeled audio datasets. By annotating audio recordings with transcriptions, speaker identifications, emotions, or other linguistic aspects, NLP models can be trained to better understand and process spoken language. This enables applications like speech recognition, sentiment analysis, virtual assistants, and voice-controlled systems to perform more accurately and effectively.
- Enabling Speech-related Applications:
Audio annotation facilitates the development of various speech-related applications. By labeling audio with speaker identities, diarization, and transcription, it becomes possible to build systems that can identify and track speakers in conversations, convert spoken words into written text, and improve speech-to-text applications. These applications find use in transcription services, voice assistants, call center analytics, and more.
- Improving Emotion Analysis:
Emotion recognition through audio annotation allows the detection and classification of emotions expressed in spoken language. By labeling audio with emotional attributes, researchers and developers can train machine learning models to identify sentiments such as happiness, sadness, anger, or neutral states. This has applications in sentiment analysis, customer feedback analysis, voice-driven emotion-aware systems, and human-computer interaction.
- Enriching Audio Content:
Annotations add valuable context and structure to audio content, making it more accessible and searchable. By transcribing audio into text, audio content becomes searchable and indexable, making it easier to retrieve specific information from large audio collections. This enhances accessibility for individuals with hearing impairments and improves content management and organization in fields like journalism, podcasting, and audio archives.
- Supporting Audio Classification:
Audio annotation enables the classification of audio into different categories based on its content. This is valuable in various applications such as acoustic data classification, environmental sound classification, music classification, and natural language utterance classification. Classifying audio allows for targeted analysis and enables systems to recognize and differentiate between different audio types, environments, or linguistic variations.
Generally speaking, audio annotation is important as it empowers NLP systems, enables speech-related applications, enhances emotion analysis, enriches audio content, supports audio classification, and facilitates the training of machine learning models. By adding annotations to audio data, we can unlock its full potential, enabling machines to better understand, interpret, and utilize spoken language in various domains and industries.
Application of audio annotation
- Call Centers and Customer Service:
Audio annotation is crucial for analyzing customer interactions and improving call center operations. By annotating call recordings with speaker identification, sentiment analysis, and intent labeling, companies can gain insights into customer satisfaction, agent performance, and identify areas for improvement in customer service.
Healthcare and Medical Research:
In the healthcare industry, audio annotation can be used for transcribing medical dictations, annotating patient consultations, or identifying specific medical terms or conditions within audio recordings. This supports accurate medical documentation, research analysis, and clinical decision-making.
Market Research and Voice of Customer (VoC) Analysis:
Audio annotation enables market research firms to analyze consumer feedback from recorded interviews, focus groups, or survey responses. By annotating audio data with sentiment, topic, or demographic information, companies can extract valuable insights and trends, leading to better product development and marketing strategies.
Media and Entertainment:
Audio annotation plays a crucial role in media and entertainment industries. By annotating audio with music genres, artist names, or lyrics, platforms like streaming services can personalize recommendations and create curated playlists. Additionally, audio annotation helps in media content indexing, facilitating efficient search and retrieval of audio segments.
Automotive and Autonomous Vehicles:
Audio annotation is essential for developing speech recognition systems within vehicles. By annotating audio with voice commands, navigation prompts, or driver-speech data, automakers can create intuitive voice interfaces that enhance driver safety, convenience, and hands-free interaction.
Security and Surveillance:
Audio annotation aids in security and surveillance applications by enabling the analysis of audio recordings for detecting specific sounds or events. Annotations can include identifying gunshots, alarms, or keywords in audio streams, helping security systems quickly identify potential threats or unusual activities.
E-learning and Language Training:
Audio annotation is valuable in language learning platforms and e-learning environments. By annotating audio with pronunciation guides, language-specific features, or phonetic transcriptions, learners can practice and improve their language skills with accurate feedback and guidance.
These applications have highlighted how audio annotation is leveraged across various industries to extract valuable insights, improve efficiency, enhance customer experience, and drive innovation in specific domains. Therefore, it is undeniable that the ability to annotate and understand audio data can bring immense value and open up new possibilities in industry-specific applications.

Applications of audio annotation
How to implement audio annotation?
To implement audio annotation effectively, you may need to follow these steps:
- Define annotation guidelines:
Before you begin annotating audio data, it’s essential to establish clear annotation guidelines. These guidelines should outline the specific information you want to annotate, such as speech segments, speaker identification, or specific acoustic events. Besides, it also needs to include the information of annotation categories and labels, and provide examples to ensure consistency among annotators.
- Preprocess the audio data:
Moreover, preprocessing your audio data can enhance the annotation process. For example, you can first convert audio files to a suitable format, such as WAV or MP3, depending on the requirements of your annotation tools. Or you may find it necessary to normalize the audio levels to ensure consistent volume across different files.
- Choose an annotation tool:
There are various options available, ranging from specialized software to general-purpose tools. Some popular audio annotation tools you can come to like Praat, ELAN, or TagOn. To have the most suitable decisions, make sure to explore their features, user interfaces, and compatibility with your operating system to make an informed choice.
- Start the annotation process:
After finishing all those aforementioned suggestions, you now may need to begin annotating the audio data according to your defined guidelines. Firstly, load an audio file into the annotation tool and start listening to the audio. After that, you can use the provided tools to mark and annotate different segments based on the predefined categories and labels.
- Review and revise annotations:
After annotating an audio file or a set of files, review your annotations for accuracy, consistency, and adherence to the defined guidelines. Check for any missed segments or errors. It can be helpful to have a second annotator or an expert review your annotations for quality assurance purposes.
By following these steps, you can implement audio annotation efficiently and produce high-quality annotated data for your research, development, or analysis tasks.
How to improve audio annotation quality?
Improving the quality of audio annotation is crucial for generating accurate and reliable data that drives the development of robust AI systems. Here are some effective strategies to enhance the quality of audio annotation:
- Having Clear Annotation Guidelines
To ensure a comprehensive workflow as well as high quality of annotated audio, it is important to develop detailed and unambiguous annotation guidelines that provide clear instructions to annotators. These guidelines may need to include the criteria for labeling different aspects of the audio, such as speaker identification, sentiment analysis, or specific linguistic features to ensure consistency and accuracy in the annotations.
- Expert Training
Besides guidelines, it is also necessary to provide thorough training to annotators, especially when working with domain-specific or complex audio data, familiarize them with the annotation guidelines, common challenges, and best practices to make sure they can meet the requirements for the project.
- Quality Control Measures
Implementing a robust quality control process to validate the annotations is also worthy to mention. What you need to do is regularly review and audit the annotations to ensure compliance with the guidelines as well as provide feedback and guidance to annotators to address any issues and improve their performance. It is also recommended to continuously evaluate the performance of the annotation process and benchmark it against industry standards.
- Utilize Technology
To improve your audio annotation, you should also leverage technological advancements to improve annotation quality. You can explore automated or semi-automated annotation tools that can assist in tasks such as speaker diarization, speech-to-text transcription, or sentiment analysis. These tools can help reduce manual effort and potential errors, improving efficiency and accuracy.
- Prepare a streamlined process
To improve audio annotation quality and encourage collaboration you also need to prepare a streamlined process. Begin by selecting representative samples for annotation to manage the workload effectively, you then may find it necessary to conduct a pilot test with a subset of samples and gather feedback to refine the annotation guidelines. After that to ensure audio annotation quality, it is a must to provide comprehensive training to annotators, fostering an environment where questions and clarifications are encouraged. Schedule regular meetings also play important roles to address questions, challenges, and provide feedback, promoting open communication. By following this streamlined process, you can enhance the quality of audio annotations and create a collaborative environment that fosters effective communication among annotators.
By implementing these strategies, you can elevate the quality of audio annotation, ensuring the generation of high-quality data that fuels the advancement of AI systems across various industries.
How does TagOn Help?

TagOn platform to boost the audio annotation project
TagOn is an advanced audio annotation platform that significantly aids in improving the quality and efficiency of the annotation process. With its comprehensive features and capabilities, TagOn offers several benefits:
- Streamlined Annotation Workflow:
TagOn simplifies the annotation workflow by providing an intuitive interface for annotators to label and annotate audio data. Its user-friendly design allows for seamless navigation and easy access to annotation tools, ensuring a smooth and efficient annotation process.
- Customizable Annotation Guidelines:
TagOn enables the creation of customizable annotation guidelines tailored to specific project requirements. Annotators can refer to these guidelines while labeling different aspects of the audio, ensuring consistency and adherence to project-specific criteria.
- Collaboration and Communication:
TagOn facilitates collaboration and communication among team members involved in the annotation process. It provides features for discussions, comments, and sharing of insights, allowing annotators to seek clarifications, exchange ideas, and address challenges, thereby enhancing the overall annotation quality.
- Quality Assurance and Validation:
TagOn includes built-in quality assurance mechanisms to maintain high annotation standards. It allows for the implementation of inter-annotator agreement checks, ensuring consistency among annotators. Additionally, the platform supports validation and auditing processes to review and validate the annotations for accuracy and reliability.
- Advanced Annotation Tools:
TagOn offers a range of advanced annotation tools to cater to diverse annotation needs. These tools may include speaker diarization, sentiment analysis, intent labeling, transcription, and more. By leveraging these tools, annotators can efficiently annotate audio data with precision and accuracy.
Summary
To sum up, audio annotation is crucial across industries, enabling speaker identification, transcription, emotion recognition, and audio classification. Its applications span across industries such as customer service, healthcare, market research, media, automotive, security, and language training, enabling businesses to analyze customer interactions, improve healthcare documentation, gain consumer insights, enhance user experiences, ensure security and surveillance, and facilitate language learning. To improve quality, you will need to establish clear guidelines, provide training, implement quality control, collaborate, and leverage technology.
With the help of TagOn, an advanced audio annotation platform that streamlines the annotation workflow, improves annotation quality, and enhances collaboration among annotators, you can optimize the annotation process, generate high-quality annotated data, and accelerate the development of robust AI models.
For more advice, please contact us at the following information:
Contact information:
Website: https://tagon.ai/en
Linkedin: https://www.linkedin.com/company/tagon-data-labeling
Facebook: https://www.facebook.com/TagOnGlobal/
Phone number: +84 2466 603 178
Email: contact@tagon.ai